The Essential Guide to Machine Learning Labeling Tools
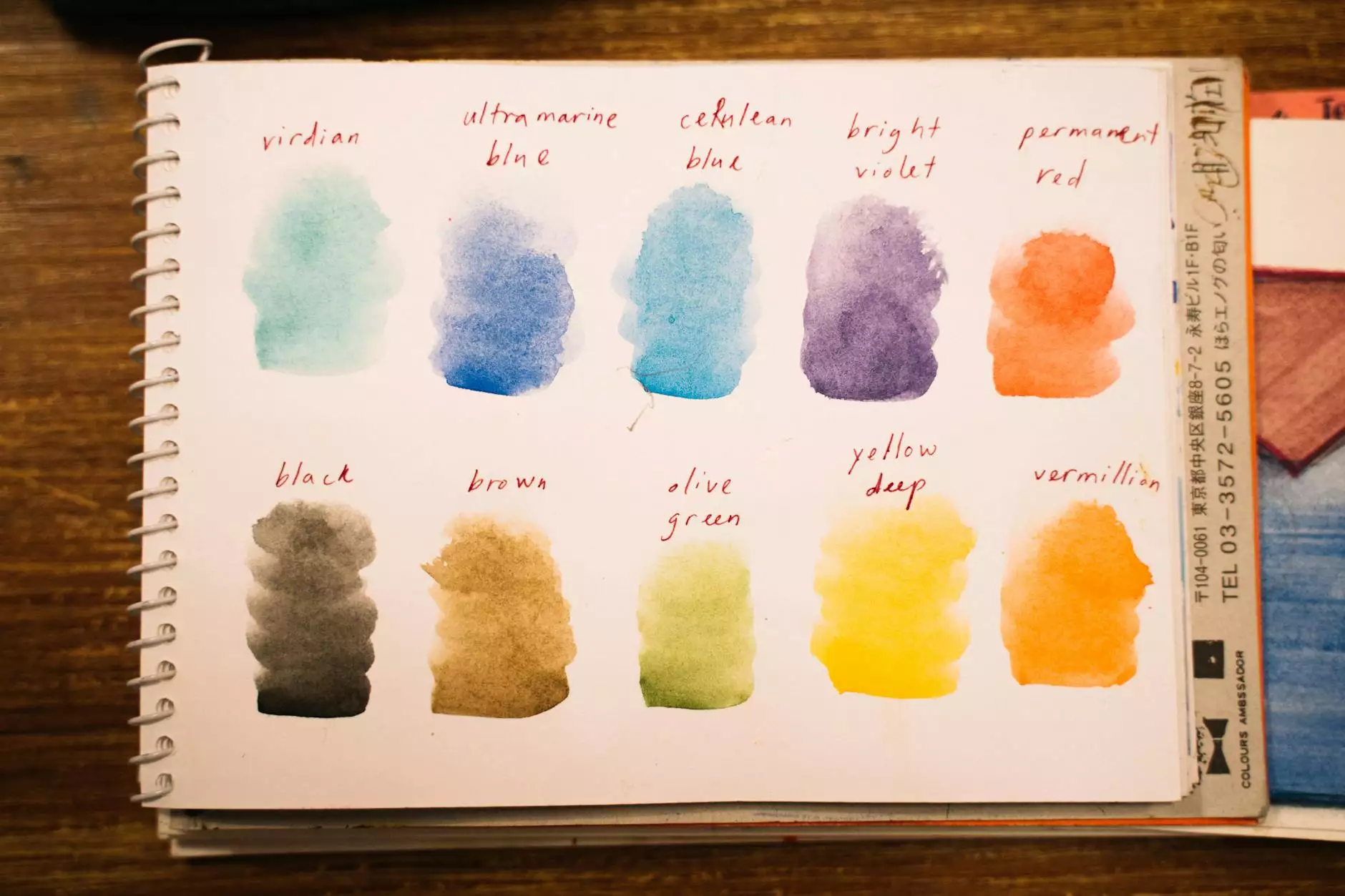
In today's data-driven world, the effectiveness of artificial intelligence (AI) solutions hinges on the quality of training data they are provided. To achieve unparalleled performance, one of the most critical aspects is the use of a machine learning labeling tool. This article delves deeply into the intricacies of data annotation, providing insights into why these tools are essential for any AI-driven project.
Understanding Data Annotation and Its Significance
Data annotation involves the process of labeling or tagging data, which allows machine learning algorithms to understand and learn from the datasets. This is a prerequisite for supervised learning. Without proper annotation, the datasets are essentially meaningless for training AI models. However, the extensive array of formats, including text, images, and videos, requires diverse annotation techniques, underscoring the importance of using an efficient machine learning labeling tool.
Types of Data Annotation
- Image Annotation: Tagging images with bounding boxes or segmentation masks to ensure machine learning models can identify objects.
- Text Annotation: Classifying text data through techniques such as entity recognition or sentiment analysis.
- Video Annotation: Tagging frames in video data for tasks such as activity recognition or object tracking.
- Audio Annotation: Labeling audio clips for speech recognition or sound classification.
What is a Machine Learning Labeling Tool?
A machine learning labeling tool is designed to assist in the data annotation process, making it easier and more efficient to prepare datasets for machine learning applications. These tools often come equipped with a range of features that enable users to annotate data with precision while minimizing potential errors. Key features typically include:
- Automated Labeling: Utilizing algorithms to suggest annotations based on learned data.
- Collaboration Tools: Allowing teams to work together seamlessly on data annotation projects.
- Quality Control Measures: Implementing verification steps to ensure data accuracy and reliability.
- Support for Multiple Data Formats: Handling various types of data inputs, including images, video, text, and audio.
Importance of a High-Quality Machine Learning Labeling Tool
Investing in a high-quality machine learning labeling tool is crucial for several reasons:
1. Improved Efficiency
Using the right labeling tool streamlines the annotation process, saving significant time and effort. Automation features often expedite the labeling process, allowing data scientists to focus more on model development rather than data preparation.
2. Enhanced Data Quality
High-quality machine learning labeling tools come with built-in checks that help improve the labeling accuracy. Features like consensus labeling, where multiple annotators provide input on the same data, can drastically reduce errors.
3. Scalability
As the volume of data skyrockets, scalable solutions are vital. A robust machine learning labeling tool can easily adapt to increasing data demands without a drop in quality or performance.
4. Cost Effectiveness
By reducing the time taken for data annotation and ensuring high accuracy from the start, businesses can save money in the long run. This financial efficiency can be especially critical for startups or businesses exploring AI solutions.
Key Features to Look for in a Data Annotation Tool
When choosing a machine learning labeling tool, consider the following features that ensure you select a platform that aligns with your project goals:
1. User-Friendly Interface
An intuitive interface enhances the user experience and ensures that even non-technical team members can participate in the annotation process effectively. This bridges the gap between technical analysts and domain experts.
2. Comprehensive Annotation Types
The tool should support a variety of annotation types to cater to different data formats, ensuring versatility across various projects.
3. Collaborative Work Environment
In highly dynamic teams, having features that allow for collaboration can significantly improve efficiency. Tools that support multiple users working on the same dataset can foster communication and improve the workflow.
4. Quality Assurance Features
Look for a tool that includes features like duplicate detection, data validation, and performance metrics to maintain high-quality standards across the dataset.
Use Cases of Machine Learning Labeling Tools
Various industries utilize machine learning labeling tools to improve their AI applications. Here are some notable use cases:
Healthcare
In healthcare, machine learning is leveraged for diagnostics and treatment suggestions. Accurate annotation of medical images (like X-rays or MRIs) is crucial for training models to identify conditions like tumors or fractures.
Automotive
The automotive industry employs machine learning for autonomous vehicle technologies. Labeling road conditions, objects, and traffic signs enhances the vehicle's ability to navigate safely.
Finance
Machine learning in finance aids in fraud detection and risk assessment. Text and transaction data labeling allows financial institutions to train models to flag suspicious activity.
E-commerce
E-commerce platforms utilize machine learning for personalized recommendations. Annotating products and user interactions enables systems to suggest products that resonate with individual customers.
Challenges in Data Annotation and How to Overcome Them
Despite the advancements in machine learning labeling tools, challenges still exist:
1. Balancing Quality and Speed
Sometimes, the rush to label data can compromise quality. Establishing a balanced workflow and using quality assurance tools can help mitigate this issue.
2. Subjectivity in Annotations
Different annotators may have varying interpretations. Implementing clear guidelines and utilizing consensus labeling can help standardize interpretations across the team.
3. Data Privacy Concerns
Data privacy is a critical issue, especially with sensitive information. Ensure that the labeling tool complies with all relevant regulations and best practices to protect consumer data.
Conclusion: The Future of Machine Learning Labeling Tools
As technology continues to advance, the demand for robust, efficient machine learning labeling tools will only grow. Innovations in AI and machine learning will continue to shape the future of data annotation. Keylabs.ai is at the forefront of this industry, offering state-of-the-art solutions tailored for various data annotation needs.
Choosing the right machine learning labeling tool is pivotal in ensuring that your AI applications achieve their full potential. Whether you are in healthcare, finance, automotive, or any other industry looking to harness the power of AI, investing in quality data annotation tools can lead to significant improvements in accuracy and efficiency, ultimately driving better business outcomes.
Explore the cutting-edge offerings at Keylabs.ai to find the perfect machine learning labeling tool that equips your business for future successes in the AI domain.